23. May 2024 By Dr. Michael Hartmann
AI - but how? 4 steps to an AI application
Machine Learning has been a central topic in the insurance industry for several years, but with the launch of ChatGPT at the end of November 2022, AI and Generative AI (GenAI) in particular has really picked up speed again. GenAI is now discussed in almost every customer conversation. This raises the questions: "Is the insurance industry ready for GenAI?" as well as "How do I go about launching a GenAI project?" We want to shed light on both these questions.
AI is revolutionizing the working world
Generative AI can be used to create texts, images, videos, analyses, programs, and more. And the beauty of it is that no complex syntax is required. iInstead, the query or instruction (prompt) can be written in natural language and passed to the AI system for interpretation. So it's no wonder that the euphoria is very real, and companies want to benefit from these new opportunities as quickly as possible. But how well prepared is the industry for the introduction of AI applications? In a study commissioned by adesso at the end of 2023, Swiss companies were asked this very question.
An excerpt of the responses is shown in Figure 1.
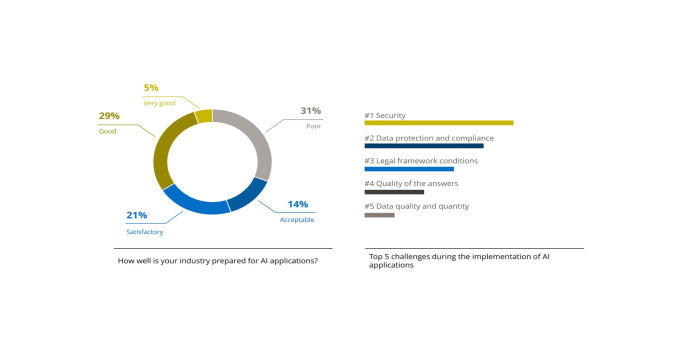
The answers of the participants regarding their readiness for AI applications can be divided into three virtually equal segments: 31 percent state that they are not yet sufficiently prepared, 35 percent assume that they are sufficiently prepared, and 34 percent believe that they are well to very well prepared. The results suggest a rather positive picture with regard to the ability to create and introduce AI applications. Admittedly, we find this result a little surprising. This is because the same survey also asked about the biggest challenges when introducing AI applications. These findings indicate that companies consider security risks, compliance and legal framework conditions as well as the quality of data input and output to be the top five challenges. All of these issues are tasks that cannot be solved on the fly, but require a careful, cross-company approach. Based on our experience, we would question the claim that the majority of these challenges have already been solved in two thirds of companies.
4 steps to the AI application
adesso has been involved in numerous AI projects and has used this experience to develop a four-step procedure for successfully introducing AI applications (Figure 2).
Step 1:
The AI strategy lays the foundations for the further development and expansion of activities. This takes place at the start of activities and involves all stakeholder groups. Typical questions that are dealt with in the AI strategy are:
- Objectives: The central question is, of course, which goals are to be achieved with AI. On the one hand, objectives can be in the direction of increasing efficiency and effectiveness, on the other hand in the direction of expanding the range of services (new products). An underlying business case with measurable KPIs must always be defined.
- Areas of application: Another point that needs to be clarified in the strategy phase is the planned area of application. The key question is whether the AI application will only be used within the company or whether there are also areas in which customers or partners will use the application. The result is a prioritized long list of use cases.
- Data sources: Every AI application is based on data. Data is therefore the central key to successful AI implementation. When considering the AI strategy, it must therefore be determined whether only internal data or also external data sources should be accessed and whether these should even be used in combination.
Step 2:
The AI architecture builds on the results of step 1. In particular, the architecture describes which data is mapped in which systems and which tools are used.
- Data storage: The platform or platforms on which data is stored and which tools access it via which interfaces must be defined.
- Data availability: Depending on the use case and area of application, AI places different demands on data storage. For example, performance, versioning or historization, standardization, consolidation and quality assurance must be checked.
- Applications: Ultimately, new tools usually have to be procured. As the market already offers a wide range of products, it is important to carry out a thorough evaluation of your own requirements in order to ensure long-term investment security.
Step 3:
In the third step, it is essential to examine which regulatory requirements need to be taken into account, particularly from a data protection and compliance perspective.
- Data protection: Just because data is available does not mean that it can be used for any purpose. In particular, if personally identifiable information (PII) is involved, strict requirements from the revised FADP and, under certain circumstances, the GDPR apply. This regulation also covers any automated case-by-case decisions if AI applications are integrated into process automation.
- Compliance: In addition to data protection requirements, there are further regulations for AI applications that will soon be adopted. Of particular relevance here is the EU AI Act, which sets out various rules for dealing with AI applications, depending on the level of risk posed by the AI system. It is currently unclear to what extent Switzerland will follow these rules and whether it will demand stricter regulation.
- Data security: Data security must always be checked when setting up new systems. The check is carried out with regard to the three protection goals of confidentiality (access authorization), integrity (protection against manipulation) and availability (resilience).
Step 4:
The first three steps lay the foundations for embedding an AI application in a company in the long term. In the fourth, the AI application is finally developed and launched. The following points should be taken into account:
- Expectation management: An AI application is a learning system that continuously develops through "learning" the more it is used. It is trained with training data, which is usually not sufficient at the beginning to exploit its full potential. Consequently, expectations must be clearly communicated so that the first experiences (moment of truth) do not lead to disappointment.
- MVP approach: It is advisable to launch an AI application as a minimal viable product (MVP) and develop it further in agile iterations. This reduces undesirable developments and allows the organization to slowly familiarize itself with the application.
- Quick wins: It is advisable to start with simpler AI applications in order to quickly celebrate the first successes and prevent resistance and motivation blockages. As every AI application should also realize a business case, an initial cost-benefit review can also be conducted quickly and serve as an argumentation aid for further expansion.
Use cases for the insurance industry
The question now arises as to where AI can be used at all in the insurance value creation process. The general answer to this question is simple: everywhere.
Figure 3 below shows examples of individual possible use cases for each core insurance process.
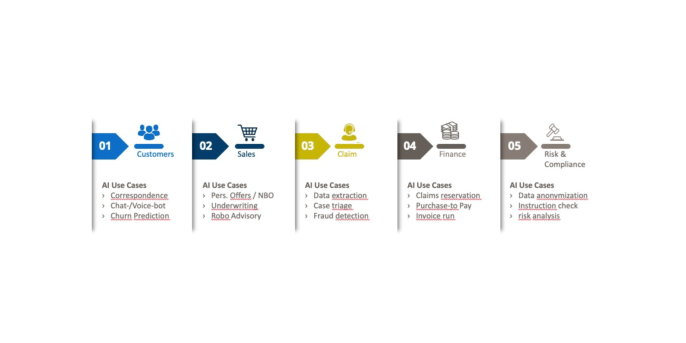
If we start with inventory management, there is already enormous potential in this first process step. For instance, all correspondence received via various channels from email to social media or chats can be analyzed, structured and processed automatically. There is further potential in the use of GenAI in customer communication (chat or voicebot) or in predictive analytics in the sense of AI-supported churn management. If we look at the next process step, "sales", there are also many opportunities for AI support here. The use case of quote personalization and next best action (NBO) is almost obvious, as much more data about the customer can be analyzed and processed promptly with AI support. However, individualized risk assessment in underwriting or even fully autonomous customer advice - as it is already being used in the financial sector - represents enormous further potential. In claims management in particular, we see great potential for automation through AI. On the one hand in case processing through data recognition and processing, and on the other through automated data interpretation and thus efficient and autonomous case triage. Predictive analytics can also be used in claims management, particularly when it comes to detecting anomalies and identifying potential insurance fraud.
Financial management also benefits from possible AI use cases. An important area of application is the risk analysis of claims, which uses AI to enable a more precise assessment of the amount of loss and the resulting need for loss reserves. We see further potential uses for AI in finance in supporting the invoice process and in the procurement process (purchase-to-pay), in which sub-processes can be handled completely autonomously. Last but not least, AI can also be used in the area of legal & compliance. The new data protection legislation in particular places high demands on the processing of personally identifiable information (PII). With the help of AI, such data can be automatically recognized and anonymized. GenAI can also be used to query or check the diversity of directives and guidelines, or predictive analytics can be used to create risk analyses and assessments at portfolio level.
Conclusion
AI is currently one of the hot topics in the insurance industry and many companies want to integrate AI into their business processes. However, they face a variety of challenges that should not be ignored. The variety of possible use cases across the entire value chain of insurers shows great potential, on the one hand in terms of automation and efficiency, and on the other hand in terms of accuracy and individualization. For these reasons, we believe it is essential not to simply start with AI use cases wherever an opportunity arises, but to choose a structured and careful approach that enables a sustainable expansion of the AI landscape.