Abstract mind games won't help you see AI’s potential. But our use cases will. Take a look at what AI is already changing here and now - in sales, in business, in service, and soon in your company. The real-world examples will give you a sense of where you can profitably use AI applications in your business.
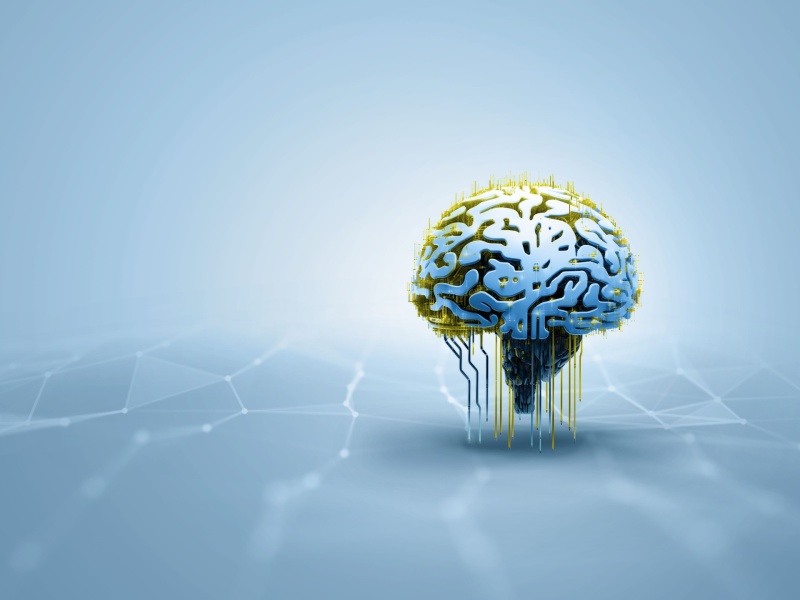
Five applications of AI in the here and now
From chatbots to lead engines
Get out of PowerPoint, into the real world.
-
A workshop that reveals the AI potential in your processes.
How it used to work:
Should we use a chatbot in service? Would we rather develop a voice solution integrated into smart speakers for ordering? Or are our customers more persuaded by hyper-personalized, automatically generated content?
Companies can use AI technologies in various application scenarios. Choosing the right approach and the right solution is critical to the success of AI projects. Those involved still lack the experience to properly assess individual application scenarios and technology potential. There is a risk that decisionmakers don’t focus on the right AI topics.
This is where the adesso workshop comes into play:
Workshops in our Interaction Room (IR) are the key to developing AI use cases. IR is a project method developed at the University of Duisburg-Essen in Germany. With the help of this method, participants can visualize interrelationships and identify risks, effort and value drivers in projects.
Together with our IR and AI experts, we identify use cases customized to your company and your goals. Regardless of whether your focus is on optimizing existing business processes, expanding your business model, or tapping new business potential, IR adapts to your current situation.
From initial ideas to analyzing existing data sources to designing and prioritizing use cases - thanks to the tried-and-tested workshop concept, we need just two days to show you in which direction you should further develop AI in your company.
This is how it is now:
From understanding the potential to integrating AI applications into existing processes and IT structures: at the end of the workshop, participants have an understanding of the opportunities that AI opens up for them.
Who is this approach suitable for?
Any company looking for AI applications to benefit its business
Who in the company is an AI application of interest to?
Anyone involved in AI projects, especially sales, marketing, customer service and IT.
-
An AI that knows how many people will call your service tomorrow - or next month
How it used to work:
Employee scheduling is one of the critical factors in service hotline quality. Too few staffed positions result in waiting times and dissatisfied customers and too many unnecessary costs. The unknown quantity is the number of calls which will be received in a day. Until now, companies relied on the gut feeling of those in charge or simple averages for this estimation - with all the unreliability and fluctuating quality that entails.
That's when AI comes into play:
Optimizing phone service capability by more accurately predicting the number of callers is a task that AI applications can comfortably deal with. Big data, a clearly defined task - and a simple criterion for measuring success: the AI-based estimates must be superior to previously employed methods.
The data basis is the caller numbers over recent years. On this basis, a machine learning system looks for patterns and finds correlations between the number of calls and factors such as the day of the week, time of day, vacation time, national holidays, weather, and advertising activities. The forecast of busyness on the service hotline is continually compared with real values and the parameters are adjusted accordingly.
How it is now:
Customers reach service staff faster and resolve their inquiries more swiftly. This ensures greater satisfaction and reduces the likelihood of switching. At the same time, work organization with machine learning provides a more reliable planning basis for both employees and managers in the call center. This helps, for instance, with effort-oriented vacation planning.
Who is this approach suitable for?
Any company with their own service hotlines.
Who in the company is this of interest to?
Anyone responsible for customer service and marketing.
For the technically interested:
- Regression problem with weak time dependency
- Standalone solution with minimal integration effort into the existing IT-landscape (communication exclusively via CSV files)
- White-box model (regression tree): This model ensures that stakeholders understand the decision-making process of the AI solution
-
An AI that translates unstructured letters into structured information
How it used to work:
The application by fax, the claim notification along with the supporting documents by letter: paper is an indispensable part of the correspondence between the insured and the insurance company. The information relevant to the processing of the case is buried somewhere in the documents: address data, contract numbers, transaction numbers, even relevant technical justifications and references. Finding this data in multiple letters, for example from different lawyers, and transferring it to an IT system is a monotonous and error-prone manual process. It ties up employee time - time they don't have to deal with complex processes.
That's when AI comes into play:
Automatically extracting structured information from unstructured texts is a typical task for AI solutions. We have developed the Heuristic Claims Management (HCM) solution specifically for use in insurance companies. The application automatically searches incoming correspondence for relevant information, prepares it and makes it available to the relevant systems for further processing in a structured form and in the appropriate formats, e.g. XML or JSON.
The data is loaded fully automatically into the processing system, manual input is eliminated, and further processing is completely digital.
How it is now:
HCM helps to avoid media discontinuities, increase processing speed and reduce error rates. The automatic processing of even unstructured information through the use of HCM helps companies achieve set digitization goals. Clerks are freed from routine activities and can concentrate on more demanding tasks.
Who is this application suitable for?
Insurance companies, banks and anyone who has paper-based processes with manual information extraction.
Who in the company is this of interest to?
- Anyone responsible for claims processing or legal protection
- Anyone involved in process automation and digitization of paper-based processes
For the technically interested:
Combination of machine learning techniques such as Support Vector Machine, Random Forest and various Bayesian analyses.
-
An AI that automates your written correspondence
How it used to work:
Any time a customer contacts a company is a valuable opportunity to check whether the quality of service advertised in marketing actually holds up. Companies invest large sums of money to meet customer expectations of individual communication as well as possible, as the service area is the flagship and contact point for your customers. Processing inquiries quickly and to the customer's satisfaction is a time-consuming and resource-intensive task. In the worst case scenario, your staff may even be distracted from their primary day-to-day tasks by processing incoming correspondence. Instead of pursuing their professional activities, they read texts, recognize the issue and delegate the tasks to the responsible colleagues. These colleagues then take over communication with the customer and formulate appropriate responses.
This is when artificial intelligence comes into play:
An AI-supported system can automate large parts of this communication process. First, the software analyzes incoming messages from various sources. It automatically recognizes important metadata such as sender information, customer or invoice numbers, and can use this to categorize the information. The application identifies the content of the letter as a decisive factor: What is the sender's concern? What answers are they looking for? Based on this text analysis, the application determines the technically correct modules in a company-specific database and independently creates individual replies.
The selection and quality of the response modules defines the quality of the responses created in this way. A team of service employees maintains and expands this database on an ongoing basis. Instead of dealing with individual cases, they use their expertise to sustainably optimize service quality across all channels.
How it is now:
Customers and interested parties receive meaningful and technically correct answers to their inquiries, and the processing time is shorter than ever. Thanks to centrally managed response modules, consistent communication is ensured across all channels. In the background, the self-learning system continuously improves the accuracy and thus the quality of the answers.
Who is this approach suitable for?
All companies with extensive customer correspondence
For whom in the company is this application of interest?
Anyone responsible for marketing, customer service, sales and distribution.
For the technically interested:
- Cognitive Services
- Azure Machine Learning
- Logic Apps
- Azure Functions
-
An AI that searches for new prospects and sales leads for you - fully automatically
How it used to work:
In the B2B environment, salespeople spend an extensive amount of time researching information: Where are potential customers planning new openings? What changes are there in management? Who has made which statements about strategic plans or investment decisions? Sales experts use these and similar topics to derive points of contact: The more customized this contact, the greater the likelihood that potential prospects will engage in the dialog. This knowledge is fundamental to sales success, but each individual salesperson must invest a lot of time in searching for information.
That's when AI comes into play:
For the corporate customer sales division of a large German bank, adesso has built an AI-based lead engine based on Microsoft. The system uses external data sources such as websites, social media content, search engines or paid corporate databases. Based on search queries or terms that indicate potential sales opportunities, the system automatically searches all connected sources. The application cleanses the results of off-target hits and duplicates using automated text analysis tools.
Each sales representative has their own dashboard where the system displays all matching hits, including background information. The sales representative evaluates each individual sales impulse. This feedback ensures that the quality of the results is continuously better.
How it is now:
The system allows employees to find prospects faster and more systematically. Thanks to the automatically prepared lead information, sales staff can invest more time in establishing and maintaining contact.
Who is this approach suitable for?
Any company with B2B sales structures
For whom in the company is this application of interest?
All those responsible for sales
For the technically interested:
- Azure Logic Apps for search and text analytics
- Cognitive services as well as Azure Machine Learning for filtering results
- Evaluation with Microsoft Power BI
Do you have any questions?
Artificial intelligence is not a replacement for human discussion
Are you wondering what possibilities AI can open up in your company? Would you like to learn more about its applications and the technology? We cannot give you a pack full of stock responses, but we can share with you our specialist knowledge, our curiosity about your company and our passion for technology.
We would be delighted to talk to you.
Head of Advisory Jean-Jacques Pittet +41 58 520 97 00